This is an ongoing project where we develop deep learning algorithms capabable of classifying the chemistry of extrasolar planet atmospheres. The algorithm is called RobERt (here is the paper) and stands for 'Robotic Exoplanet Recognition'. Deep belief neural networks, or DBNs such as RobERt, were developed more than a decade ago and are commonly used for speech recognition, Internet searches and tracking customer behaviour. RobERt’s DBN has three layers of unit processors, or ‘neurons’. Information is fed into a bottom layer of 500 neurons, which make an initial filter of the data and pass a subset up to the second layer. Here, 200 neurons refine the selection and pass data up to a third layer of 50 neurons to make the final identification of the gases most likely to be present. To prepare RobERt for his challenge, we created a total of 85,750 simulated spectra, covering five different types of exoplanet ranging from GJ1214b, a potential “ocean planet”, to WASP-12, a hot Jupiter orbiting very close to its star. Each spectrum in the training set contained the fingerprint of a single gas species. RobERt’s learning progress was tested at intervals during the training with ‘control’ spectra. At the end of the training phase, RobERt had a recognition accuracy of 99.7%.
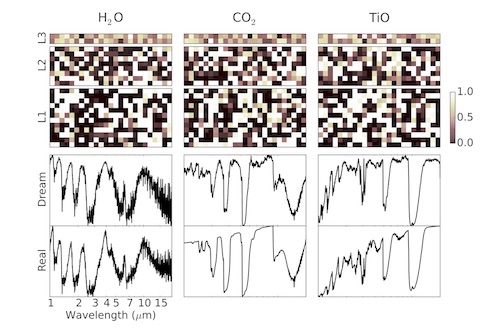
I made the top image for the Royal Astronomical Society press release. It is a neural network that was trained to re-draw pictures in the style of Claude Monet. I used this code, a water lilly painting and a photograph from Earth. The background was added later.